
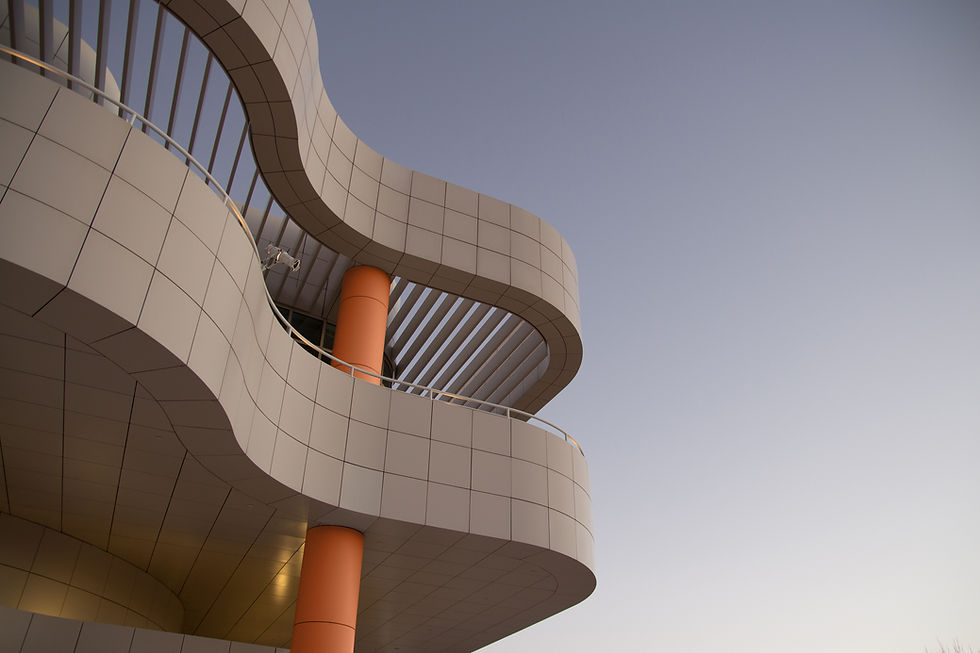
Balancing Innovation and Impact: A Realistic Look at AI’s Environmental Footprint
Mar 19
4 min read
0
2
0
By now, you’ve probably heard that AI has a major environmental impact. There have been memes and stories calling AI an “environmental disaster” and claiming that every time you make a certain number of prompts, you might as well pour a bottle of water on the ground. While those may, or may not, be overly dramatic statements, it’s important to recognize the environmental impact of AI. So what’s the truth?
Comparing AI Power Consumption to Other Sectors
AI, particularly in its training phase, is notorious for its high energy demands. Data centers that power AI models consume vast amounts of electricity – often comparable to the energy usage of small cities. For instance, while a typical data center might run continuously to support web services and cloud storage, the energy required to train a state-of-the-art generative AI model can be several orders of magnitude higher in a very short span. This stark contrast underscores that while everyday operations have their footprint, the burst of energy needed for training creates a unique challenge for sustainability. As AI gets more and more powerful, we have to hope that the increases in efficiency outpace the increases in demand for power.
Training vs. Usage: Understanding the Difference
One of the most critical distinctions in AI’s environmental impact lies between training and usage (inference):
AI Training:
Training a model involves processing enormous datasets through computationally intensive algorithms over days or even weeks. This phase typically leverages powerful GPUs or specialized hardware, leading to significant energy consumption. Although training is a one-time (or infrequent) event for a given model, the energy burst during this period can be substantial.
AI Usage (Inference):
By the time you interact with an AI, it’s already trained. Once a model is trained, it’s deployed to serve real-time queries. Although each individual inference might consume far less energy than training, the cumulative effect—especially when scaled across millions of queries—adds up. However, the overall energy footprint per query remains lower compared to the initial training phase. That doesn’t necessarily mean you should use AI guilt free – increases in consumer demand are why more and more models get trained – but it’s a valuable distinction.
Reducing the Environmental Impact of AI Training
Given the high energy costs associated with training, AI companies have a responsibility—and an opportunity—to implement sustainable practices. Some strategies include:
Optimizing Hardware and Algorithms:
Employing more energy-efficient processors and refining training algorithms can reduce energy usage without compromising model performance.
Leveraging Renewable Energy:
Transitioning data centers to renewable energy sources (like wind or solar) can drastically cut the carbon footprint associated with heavy computations. Innovative cooling solutions and energy reuse strategies can further enhance efficiency. A major demand for energy from renewable resources as part of the AI boom could be a major driver of innovation in the renewable energy field.
Collaborative Approaches:
By pooling resources or sharing best practices, companies can collectively lower the environmental burden. In many cases, sustainable practices not only reduce costs but also build a positive brand reputation.
Environmentally Conscious AI Deployment for Businesses
Businesses integrating AI into their operations can take several steps to ensure that the technology is “worth it” from an environmental standpoint:
Opt for Retrieval-Augmented Generation (RAG) Over Full Fine-Tuning:
When a fully custom model isn’t necessary, using RAG techniques can achieve high-quality outputs with significantly lower energy costs. This approach combines pre-trained models with targeted data retrieval, minimizing the need for extensive retraining. As we discussed above, the training phase is by far when energy demands are highest, so using an “out of the box” solution backed by a database rather than a custom-trained AI can be a huge savings in both financial and environmental cost.
Utilize Pre-Trained Models:
Rather than training models from scratch, leveraging pre-trained models (and adapting them with minimal fine-tuning) can conserve energy. This not only reduces power consumption but also accelerates deployment timelines.
Monitor and Optimize Usage:
Continuously track energy consumption across AI deployments and adjust operations based on efficiency metrics. This proactive approach can help businesses maintain a balance between innovation and sustainability. Whether you’re using a physical or virtual server, letting the server go idle while unused can save a fortune and keep your power demands low.
It’s important to recognize that the environmental impact of AI is not evenly distributed. Some regions bear a disproportionate share of the energy burden, a challenge that demands thoughtful policy and industry collaboration. It’s not enough to just move the problems to someone else’s backyard, we need genuine solutions.
Conclusion
The conversation about AI’s environmental impact is complex and multifaceted. While the energy demands of AI—especially during the training phase—are significant, there are clear pathways to mitigating these effects. By adopting more efficient practices, leveraging renewable energy, and choosing smarter deployment strategies like RAG, both AI companies and businesses can ensure that the benefits of AI are truly “worth it.”
Acknowledging the problem is the first step toward sustainable innovation. As we continue to push the boundaries of what AI can do, let’s also commit to making its footprint as light as possible on our planet.